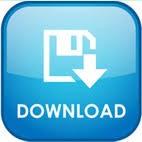
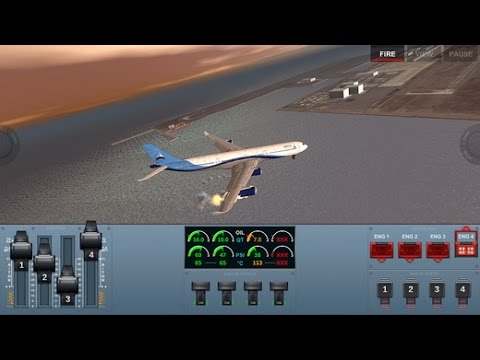
- #EXTREME LANDINGS PRO START ENGINE AFTER TURNED OFF UPDATE#
- #EXTREME LANDINGS PRO START ENGINE AFTER TURNED OFF FULL#
#EXTREME LANDINGS PRO START ENGINE AFTER TURNED OFF UPDATE#
Although modern autopilots can maintain or hold a desired heading, speed, altitude, and even perform auto-land, they cannot handle complete flight cycles automatically, and they must be engaged and operated manually by the human pilots to constantly change and update the desired parameters. In contrast, Automatic Flight Control Systems (AFCS) or autopilots are highly limited, capable of performing minimal piloting tasks.
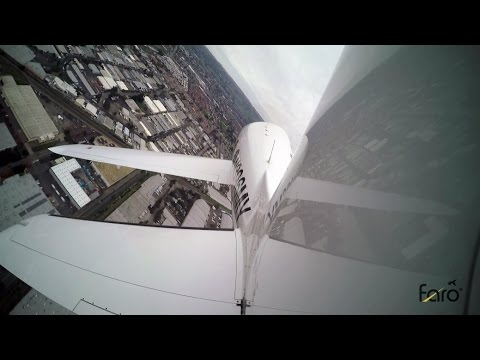
Performing a complete flight cycle starts with a ground-run on the runway to gain speed, rotate after a certain airspeed is achieved, climb, cruise while navigating between waypoints, descend, prepare for final approach while intercepting the landing runway path line, touchdown, flare, and slowdown to taxi speed. Human pilots are trained to perform piloting tasks that are required during the different phases of the flight.
#EXTREME LANDINGS PRO START ENGINE AFTER TURNED OFF FULL#
The proposed IAS is a novel approach towards achieving full control autonomy of large jets using ANN models that match the skills and abilities of experienced human pilots and beyond. In addition, experiments show that the IAS can handle landing in extreme weather conditions beyond the capabilities of modern autopilots and even experienced human pilots. Experiments show that small datasets containing single demonstrations are sufficient to train the IAS and achieve excellent performance by using clearly separable and traceable neural network modules which eliminate the black-box problem of large Artificial Intelligence methods such as Deep Learning. In addition, we introduce new ANNs trained to control the aircraft’s elevators, elevators’ trim, throttle, flaps, and new ailerons and rudder ANNs to counter the effects of extreme weather conditions and land safely. The control models imitate the skills of the human pilot when executing all the piloting tasks required to pilot an aircraft between two airports.
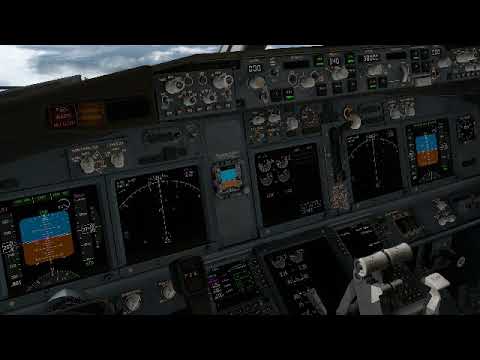
The training data is used by Artificial Neural Networks to generate control models automatically.
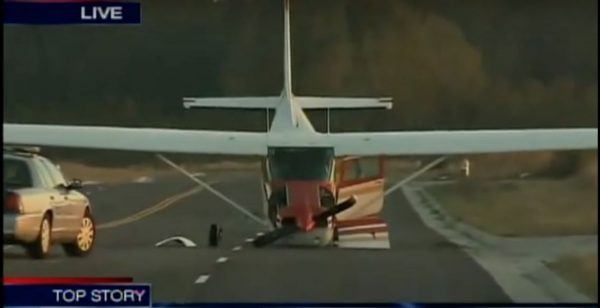
In this paper, we present the work done by collaborating with the aviation industry to provide training data for the IAS to learn from. The IAS is a potential solution to the limitations and robustness problems of modern autopilots such as the inability to execute complete flights, the inability to handle extreme weather conditions especially during approach and landing where the aircraft’s speed is relatively low, and the uncertainty factor is high, and the pilots shortage problem compared to the increasing aircraft demand. In addition, the IAS is capable of autonomously landing large jets in the presence of extreme weather conditions including severe crosswind, gust, wind shear, and turbulence. The IAS is capable of autonomously executing the required piloting tasks and handling the different flight phases to fly an aircraft from one airport to another including takeoff, climb, cruise, navigate, descent, approach, and land in simulation. We describe the Intelligent Autopilot System (IAS), a fully autonomous autopilot capable of piloting large jets such as airliners by learning from experienced human pilots using Artificial Neural Networks.
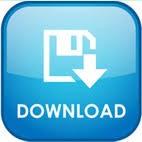